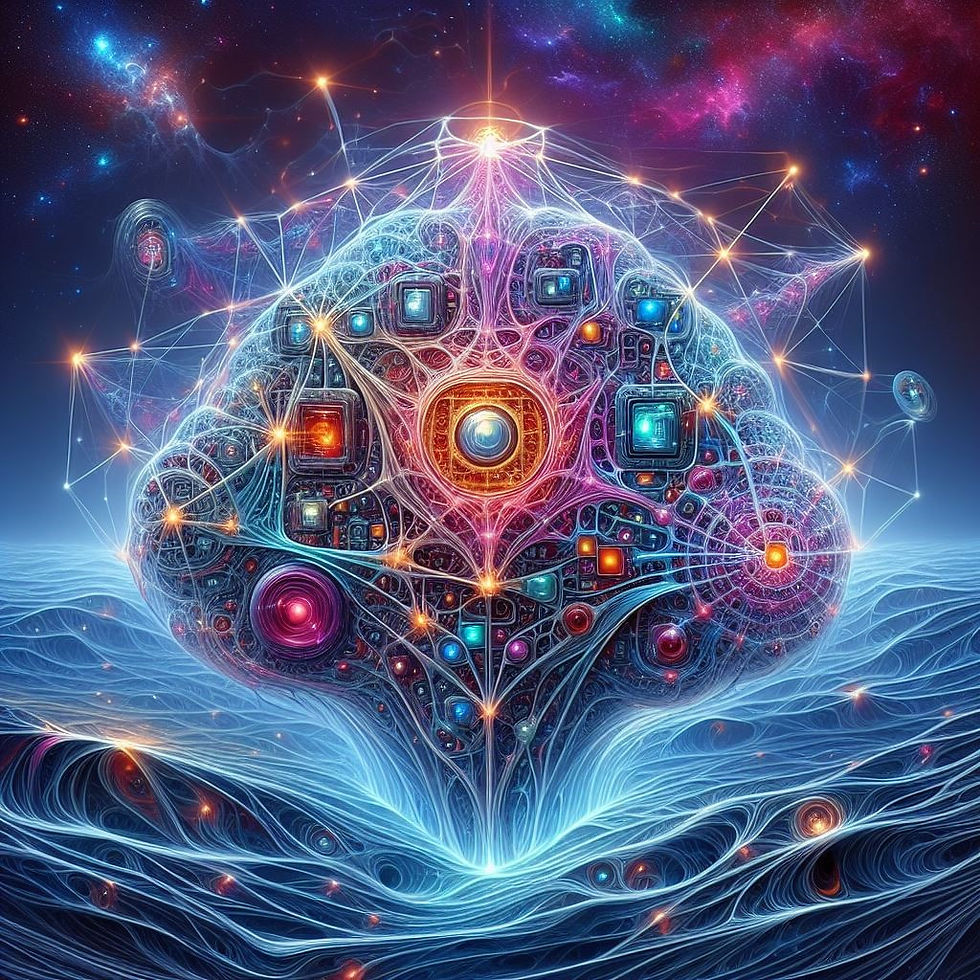
Here’s a basic example of how you might use a machine learning model (like a classifier) with Django. This example assumes you have a trained model saved as model.pkl.
First, let’s create a new Django app:
python manage.py startapp ai_app
Then, in your ai_app directory, create a new file named ml_model.py:
import pickle
from sklearn.feature_extraction.text import CountVectorizer
# Load the model and vectorizer
with open('model.pkl', 'rb') as f:
model = pickle.load(f)
vectorizer = CountVectorizer()
def classify_text(text):
# Vectorize the text
text_vector = vectorizer.transform([text])
# Predict the class
prediction = model.predict(text_vector)
return prediction
In your views.py file, you can now use this function to classify text:
from django.http import JsonResponse
from .ml_model import classify_text
def classify(request):
text = request.GET.get('text', '')
prediction = classify_text(text)
return JsonResponse({'prediction': prediction})
This is a very basic example and might need to be adjusted based on your specific use case and the type of AI model you’re using. Also, remember to add the necessary path to your urls.py file to route requests to this view.
Please note that this script assumes that you have a trained machine learning model saved as model.pkl and that you’re using a CountVectorizer for text processing. If your setup is different, you’ll need to adjust the script accordingly. Also, don’t forget to install necessary libraries such as scikit-learn and pickle in your environment.
This script also doesn’t include any error handling or input validation, which you should add in a production environment. Always ensure that user inputs are validated and errors are properly handled.
Remember to replace 'model.pkl' with the actual path to your model file. If the model file is not in the same directory as your Django project, you’ll need to provide the full path to the file.
I hope this helps! Let me know if you have any questions.
Hier ist ein einfaches Beispiel für die Emotionserkennung anhand von Gesichtsausdrücken:
Stell dir vor, du hast ein Bild einer Person und möchtest die Emotionen auf ihrem Gesicht erkennen. Ein Deep Learning-Modell, das für die Emotionserkennung trainiert wurde, könnte wie folgt arbeiten:
1. **Bildvorverarbeitung**: Das Bild wird in ein Format umgewandelt, das vom Modell verarbeitet werden kann. Dies kann das Skalieren des Bildes und das Normalisieren der Pixelwerte umfassen.
2. **Merkmalserkennung**: Das Modell extrahiert Merkmale aus dem Bild, wie z.B. die Position der Augen, des Mundes und anderer Gesichtszüge.
3. **Klassifikation**: Basierend auf den extrahierten Merkmalen klassifiziert das Modell die Emotion. Typische Emotionen, die erkannt werden können, sind Freude, Traurigkeit, Wut, Überraschung, Angst und Ekel.
Hier ist ein Beispielcode in Python,…
Das Projekt, bei dem Deep Learning-Modelle verwendet werden, um Emotionen zu erkennen und zu lernen, ist ein spannendes und wachsendes Forschungsgebiet. Hier sind einige wichtige Punkte dazu:
1. **Emotionserkennung**: Deep Learning-Modelle können trainiert werden, um menschliche Emotionen anhand von Gesichtsausdrücken, Sprachmustern und anderen biometrischen Daten zu erkennen. Diese Technologie wird oft als "Emotion AI" oder "Affective Computing" bezeichnet².
2. **Verwendung von Frameworks**: Häufig werden Frameworks wie TensorFlow und Keras verwendet, um diese Modelle zu entwickeln. Diese Frameworks bieten leistungsstarke Tools zur Erstellung und Schulung neuronaler Netze³.
3. **Anwendungsbereiche**: Die Erkennung und Interpretation von Emotionen kann in vielen Bereichen nützlich sein, z.B. im Kundenservice, im Marketing oder in der Gesundheitsversorgung. Systeme können so angepasst werden, dass sie auf die emotionalen Zustände…
Django, a high-level Python web framework, provides a powerful and intuitive way to interact with databases. Let's delve into the details:
1. Supported Databases:
- Django officially supports several databases:
- PostgreSQL
- MariaDB
- MySQL
- Oracle
- SQLite
- Additionally, there are third-party database backends that Django can work with¹.
2. Database Interaction in Django:
- Models: In Django, models serve as the single, definitive source of information about your data. They are Python classes that map to database tables and include fields, options, and methods for customization³.
- Database Queries: Django simplifies creating, accessing, and managing databases within web applications. You can make queries, perform aggregation, use search managers, and even execute raw SQL queries².
3. Connection…